Four ways to cut life science R&D costs with Design of Experiments
If you’d like to keep learning about DOE after you're done with this article check out our other DOE blogs, download our DOE for biologists ebook, and watch our DOE Masterclass Series.
Life science R&D is notoriously expensive:
-
Estimates of the average capitalized pre-launch R&D costs for a new pharmaceutical vary from US$161 million to US$4.54 billion (2019 prices)1
-
Top pharmaceutical companies invest up to 40% of their revenue in R&D2
-
The industry’s Internal Rate of Return (IRR) was down to 1.2% in 2022, lower than pre-pandemic levels3
And costs keep rising. But there is one methodology that can help organizations, laboratories, and individual researchers answer critical biological and commercial questions at a significantly lower cost. And it drives better science, too.
That methodology is Design of Experiments (DOE).
Why does this matter?
Making R&D cost-effective is critical for companies and funding bodies to keep their life-saving biological research going.
DOE can make R&D more effective (more likely to produce the desired results) and more efficient (producing desired results without wasting materials, time, or other resources). The examples of time and money saved using DOE in this blog aren’t theoretical: they’re drawn from real-life case studies.
1) DOE reduces the cost of experimentation
Experiments cost money. At an institutional level, these costs add up fast:
- Scientist time
- Equipment time
- Consumables like pipette tips, culture plates, slides, stripettes, etc.
Inefficient experimental designs drive costs in two different ways:
- Single factor/OFAT (one factor at a time): multiple runs to get limited or no results, therefore needing even more runs
- Full factorial: an enormous number of runs, likely far more than needed in an efficient DOE experiment with a tailored design (a very expensive “sledgehammer to crack a nut”)
The additional cost may not feel like much for an individual experiment or campaign. But at an institutional level, reducing the number of runs can translate into considerable savings over time.
Consider the experience of one such pharmaceutical company and customer of ours. Part of the top 20 R&D spenders, they used Synthace’s digital experiment platform to improve their assays. They compared a 672-run full factorial design with a 108-run D-optimal design. The custom D-optimal design needed 6 times fewer wells to reach the same conclusion as the full-factorial design.
Another part of the program aimed to reduce the cost of an expensive assay by using DOE to investigate a wide selection of factors to understand the drivers of the response and where expensive reagents could be minimized.
The investigation resulted in a model with two peak conditions, one of which approximately halved expensive reagent use while maintaining a similar assay quality (Figure 1).
Figure 1: Custom D-optimal design reached same conclusion as a full factorial design but with six times fewer wells
2) DOE can reduce reagent cost
Large-scale mammalian cell culture can consume huge amounts of cytokines and growth factors:
- These can be very expensive, typically US$370–860 for between 10-100µg
- Minimizing their use is a priority for companies aiming to produce large amounts of mammalian cells
Uncommon, formerly HigherSteaks is one company making lab-grown meat.
They Reprogram cells from an animal into stem cells. An animal-free growth media allows the cells to grow into muscle, fat, and other types of tissue to take the form of the meat product. But commercial growth media increased production costs at least 100-fold compared with livestock farming. In addition, common components in these media are not appropriate for human consumption.
That’s why Uncommon used DOE to accelerate media optimization.
Here are some of their goals that would have been impossible with single-factor experimentation:
- Initial factor screen
- Building an understanding of interactions
- Consistent reproducibility for continued optimization of media to direct cell growth toward a particular tissue type.
A fractional factorial DOE design allowed the Uncommon team to screen 22 factors and profile the interactions in 320 experimental runs.
Assuming two levels for each factor, a full factorial design would have required at least 4.2 million experimental runs. Using Synthace to facilitate DOE also saved the lead scientist from performing 20,000 manual calculations, AKA: a hell of a lot of time.
Using a fractional factorial DOE design, the Uncommon team got the following results:
- Campaign took a few weeks instead of 6-9 months
- Significantly increased their cellular yield
- Reduced their costs “by an order of magnitude”
3) DOE improves experiment quality
DOE experiments are more reliable and of higher quality than those obtained with OFAT:
- DOE reduces a system’s intrinsic error and means you’ll spend less time hitting experimental dead ends or chasing red herrings
- DOE offers a more complete view of the experimental space and, in turn;
- DOE gives more confidence about the results than using repeated single-factor experiments
At an institutional level, DOE fosters collaboration. Teams can, for example, systematically explore an experimental space, rather than taking a scattergun approach. This reduces the inconsistencies that usually hamper attempts to combine results.
Done right, this means that new experiments can start better informed, and experimenters need to waste less time doing similar experiments to earlier ones, saving money and time.
4) DOE improves experiment robustness
The robustness phase of a DOE campaign determines the extent to which the system is sensitive to changes in levels of factors.
A robust system will have smaller variability to changes than a less robust system.
This can be especially important when establishing that a process is robust to pharmaceutical, food, and other regulatory authorities. Here are some examples of where this could be useful:
- DOE can determine if temperature influences outcome… instead of waiting until something goes wrong with the lab’s environmental control
- DOE can be used to create processes that are inherently robust to external changes by using techniques from Quality By Design (QbD)
- DOE can be used to future-proof processes to make cost savings compared to the difficulties of re-optimizing an existing process that is perhaps vulnerable to some unknown external variation
In a further real-world example, Oxford Biomedica wanted to improve the efficiency and robustness of its in-house lentiviral vector transduction by optimizing transfection reagent mixes:
- The production process can create ineffective vector particles
- The ratios and interactions of many components contribute to the transfection process, determining the efficacy of vector particle production
- Optimizing transfection reagent mixes required a multifactorial design
The Oxford Biomedica team wanted to investigate the benefits and value of deviating from a traditional iterative DOE methodology to a quicker optimization strategy. Oxford Biomedica used DOE to design, plan and execute a two-iteration optimization and robustness study of their transfection and transduction processes (figure 2).
Figure 2: Two DOE optimization strategies. A typical DOE experimental optimization usually follows a factor screen, iterate, refinement, and optimization framework (top panel). Oxford Biomedica investigated a rapid iteration DOE strategy, with a factor screen followed by joint refinement and optimization iteration (bottom panel)
These were their results:
- Up to 10X increase in vector titer
- 81% reduction in variability
- 32% resource saving
Results like these would have been practically impossible without DOE.
DOE for cost reduction: the bottom line
We all want R&D to be more effective and less expensive. DOE offers a way to make both of these things happen, regardless of the scale or type of biological research you’re working on.
- DOE reduces experiment cost
- DOE can reduce reagent cost
- DOE improves experiment quality
- DOE improves experiment robustness
We’ve seen DOE on the Synthace platform realize these benefits in multiple applications: from assay development to media optimization to lentiviral vector transduction.
Of course, the time and resource savings arising from DOE depend on individual circumstances. But these examples offer a benchmark and show that using DOE to improve R&D cost-effectiveness is more than an aspiration. DOE’s theoretical benefits translate into the real world.
For more DOE content check out our other DOE blogs, download our DOE for biologists ebook, and watch our DOE Masterclass Series.
References
- Schlander M, Hernandez-Villafuerte K, Cheng C-Y, et al. How much does it cost to research and develop a new drug? A systematic review and assessment. PharmacoEconomics 2021;39:1243-1269
- Buntz B, Pharma’s top 20 R&D spenders in 2021, Drug Discovery & Development. Available here.
- Measuring the return from pharmaceutical innovation 2022, Deloitte. Available here.
Michael "Sid" Sadowski, PhD
Michael Sadowski, aka Sid, is the Director of Scientific Software at Synthace, where he leads the company’s DOE product development. In his 10 years at the company he has consulted on dozens of DOE campaigns, many of which included aspects of QbD.
Other posts you might be interested in
View All PostsImplementing Design of Experiments (DOE): A practical example
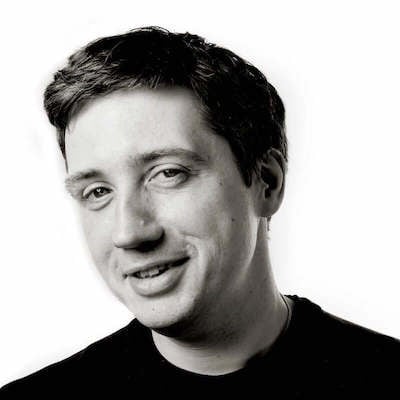
What is Design of Experiments (DOE)?
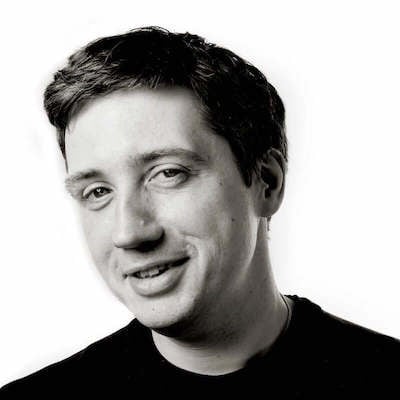
Overcoming barriers to Design of Experiments (DOE)
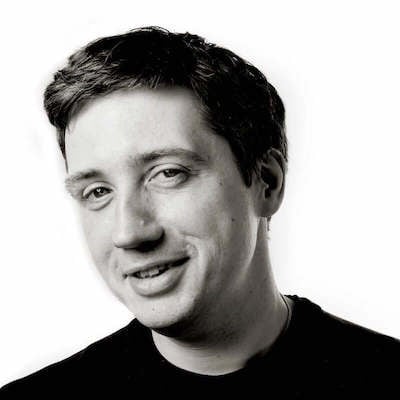