- Biologists are almost spoilt for choice when it comes to Design of Experiments (DOE) applications. This blog explores how to choose the right DOE experiment to reach your experimental goals.
- We’ll introduce the three main aims of a DOE campaign (characterization, optimization, and assessing consistency) and explore how these relate to your experimental goals.
- The blog also discusses the value of setting goals for responses and how DOE approaches can combine multiple responses.
- We will consider how to use replication in a DOE campaign to measure intrinsic variability and introduce using a model to assess robustness.
- If you’d like to read or learn more about DOE, make sure to check out our other DOE blogs, download our DOE for biologists ebook, or watch any of the webinars in our DOE Masterclass Series.
Biologists are almost spoilt for choice when it comes to Design of Experiments (DOE) applications (figure 1). As we have seen, though, DOE sometimes is an experimental sledgehammer to crack a nut of a hypothesis. This blog explores how the goals of your biological experimentation relate to the type of DOE experiment you might design or find in a busy lab.
Figure 1: Real-World Applications
Easier said than done: from eukaryotic to prokaryotic
In a previous blog, we looked briefly at the application of DOE in producing protein ‘X’ in a bacterium lineage. This time we’ll move from a prokaryotic line to a eukaryotic expression system. In this case, a yeast.
Bacteria and yeasts come from different taxonomic kingdoms. We can use the knowledge obtained from bacterial experiments to inform the conditions needed for expression in yeast. But we shouldn't expect the transfer from prokaryotic to eukaryotic lines to be straightforward.
The genetic payload will need to be at least partially rewritten, for instance. Getting a eukaryote to stably express the gene or genes of interest can be more difficult than with prokaryotic lines. The media and other growth conditions will be different. In general, the more complex organisms become, the greater the stochasticity in behavior and systems tend to get noisier. Maintaining relative stability means considering a greater number of factors and inputs compared with simpler organisms.
So, how can DOE help you meet your goal of optimal expression by the yeast?
When and how can DOE help?
Numerous factors could influence the stable expression of the gene encoding protein ‘X’ by the yeast. Fungi use several amino acids as nutrition, for example, which could be worth investigating. And that’s just one component of the complex and complicated pathways in a yeast.
In general, DOE assumes that you have a good starting point. Your results will greatly depend on where you start in general. Although we know inputs and conditions will change, in our example it's reasonable to assume our existing process is “good enough.” A future blog will look at what happens when you don’t know where to start.
In our example, we could use DOE to meet several experimental goals, such as:
- Ensuring the system meets our experimental objectives: e.g. the yeast line expresses the gene encoding protein ‘X’ with at least the same specific productivity as the original bacterial line
- Fixing a specific problem with a part of the system: e.g. expression levels of the gene vary markedly
- Better understanding without necessarily aiming for control, e.g. which balance of amino acids has the biggest effect on the expression levels by the yeast
- Adapting the process after development: e.g. scaling up or using a new, cheaper feedstock
DOE experiments can generally be bucketed into three broad objectives:
- Characterization
- Optimization
- Assessing consistency
We’ll use these classifications to illustrate how you can apply DOE experiments to achieve different experimental objectives such as the ones above.
Characterization
Ensuring stable expression of the gene encoding protein ‘X’ by the yeast can be a long experimental journey. But of course, a journey of a thousand miles begins with a single step. Characterization is about understanding the likely journey better. You’re not yet trying to find the best route.
One example of how to use characterization is in finding a starting point. Without going into too much detail (we promise to consider the nuances in another blog), some DOE designs allow you to investigate your experimental space broadly, without making too many assumptions.
In these circumstances, DOE experiments can screen a broad set of possible processes and genetic factors. This identifies experimental spaces to explore further. The DOE toolbox contains several possible approaches, although scoping and space-filling designs (figure 2) work well for this purpose.
Figure 2: scoping design
Characterization: other examples
In most cases, you do have a starting point such as the bacterial process in our example. Characterization is still useful even if you have some information. For example, DOE experiments can differentiate the most influential factors affecting, in this case, gene expression by the yeast, from those that are less influential or trivial. So, we may test the components in the culture medium to determine which make the largest contributions to gene expression and which may not be needed. This is the classic logic of the screening stage, as discussed in a previous blog.
Beyond this, characterization helps understand the effects of future possible variations. Even though we have an immediate application in mind, there will be other uses in future that will probably vary in one way or another. Understanding if processes can adapt to these in principle, future-proofs the system and saves expensive development time.
So, the base medium from company A contains a different mix of amino acids to that from company B. Does the different mix of amino acids influence gene expression? Characterizing the medium’s effect on expression is useful if you think company A’s medium (which you normally use) may be difficult to source in the future. You may also want to characterize an assay to see whether it could be adapted to future needs such as high or low pH or the presence of solvents such as dimethyl sulfoxide (DMSO).
Optimization
In most cases, you don’t want to just understand what’s going on, you also want to find a way to get your system to do something useful in the most efficient way you can (figures 3 & 4). To do this still requires you to characterize the system to some extent - it’s not possible to exert much control if you don’t have at least some understanding of what’s going on. But the experimental goal during optimization differs from characterization, which leads to a different campaign structure and use of information.
Figure 3: Construct assembly optimization
Optimizing a response is usually done in one of three ways: maximizing, minimizing, or aiming to be as close as possible to a specific value. So, in our yeast experiment, we would perhaps aim to determine the combination of components and the levels in the culture medium that maximize the expression of the target gene.
This could work well, but it’s important to think carefully about whether you’re optimizing for the right thing. Maximizing gene expression alone could give you the best productivity. But it’s quite likely that the relationship between expression and yield is quite complicated. You could add several other responses with different targets. But perhaps choosing the simplest approach and directly maximizing the yield, leaving the interplay of underlying mechanisms unspecified, would be a better choice.
Optimization: applying Constraints
In many cases, it’s less important to get strictly the highest amount of something you possibly can than to get at least some minimum amount (2 g/l yield, for example). Similarly, it’s likely that there will be a maximum amount of certain impurity you can tolerate from the process. Optimization allows us to impose constraints on responses to achieve things like this.
Returning to our example, we may start by selecting potentially interesting strains based on their ability to produce at least a certain product concentration in pilot conditions, which approximate the eventual process conditions. A threshold value of some reasonable fraction of the production achieved in the bacterial system may be a good choice.
Figure 4: incremental improvement on the assembly efficiency over the different stages
Optimization: achieving the best outcome
We can often find aggregate measures (such as in the yield example above) that avoid the need to dissect how our system does what we want in too much detail. But it’s usually necessary to include more than one response in our optimization. In technical terms, this makes our problem one of multi-objective optimization. We already touched on one version of this above, where we tried to achieve a high yield with a low level of impurities.
As another example: imagine that our medium contains several expensive components. Aiming to find conditions that maximize target gene expression by the yeast for the lowest cost would combine maximizing the expression of the gene with minimizing the cost of reagents used. In this example, the response is calculated rather than measured.
Consistency
Measuring or improving the consistency of a system when run repeatedly in real conditions is an important application of DOE techniques. One of these applications is known as Quality By Design (QbD), which is a sub-discipline of measuring or improving the consistency of a system and often taught as part of the process improvement framework Six Sigma. There are two important dimensions to consider: intrinsic variability and robustness.
Consistency: reducing variability
We use variability here to refer to intrinsic variability (i.e. changes in response values that occur despite identical input conditions). For biological processes, this lack of consistency loosely breaks down into a component coming from the organism (e.g. time spent in different phases of the cell cycle or development or variable expression of promoter sequences during colony morphogenesis) and a component coming from the experimental conditions (e.g. ± 5% in the concentration of glucose and fructose or ± 0.5 pH units).
As you probably know already or could guess from the above, the statistical answer to this is to use replication in your experiments to measure the degree of intrinsic noise. You could include intrinsic noise as a response to be minimized alongside your measures of the output’s quality and quantity.
Consistency: improving robustness
Robustness refers to the system’s sensitivity to changes in its inputs. Returning to our expression experiment: in ideal circumstances, the yield (e.g. gene expression) would not significantly alter despite changes to conditions and inputs (e.g. different suppliers of the flow cytometry kit) or environment (e.g. ambient laboratory temperature). But, of course, most of these have some effect. One of the most important uses of DOE techniques is to help choose process conditions that minimize this sensitivity.
More specifically, you may want to ensure that the yeast yields the same gene expression (e.g. by ± 2.5%) independent of variations in the composition of medium (up to values guaranteed by the manufacturer). In this case, DOE experiments can target gene expression and vary the medium alongside other factors. By looking at the model coefficients for the medium and other factors as main effects and in interaction, we can aim to find conditions that produce less than 2.5% variation in expression consistently even given the noise in the input factors. A common final validation step simulates, using the derived model, given variation in the inputs to make sure that the level of output variation is within limits. QbD is a big topic that we will discuss in future blogs.
Conclusions
This blog helps bridge the gap between the way DOE characterizes and informs experimentation and the way biological scientists think. We’ve looked at the three main aims of a DOE campaign (characterization, optimization, and assessing consistency) and explored some details of how these relate to your experimental goals.
Specifically, we’ve discussed how goals for responses help you achieve your experimental goal and how to combine multiple responses. We also discussed using replication to measure intrinsic variability and introduced using a model to assess robustness. Generally, the perspectives here all relate to optimization: one of DOE’s foundations. We’ll discuss optimization further in future blogs.
If you’d like to read or learn more about DOE, make sure to check out our other DOE blogs, download our DOE for biologists ebook, or watch any of the webinars in our DOE Masterclass Series.
James Arpino, PhD
Dr James Arpino, aka JAJA, is a Product Manager at Synthace, where he leads the product development of experiment design and planning. In his seven years at the company he has become an evangelist and expert in transformational multifactorial methods in biology, including DOE.
Other posts you might be interested in
View All PostsDOE in one place with Synthace's accessible workflow
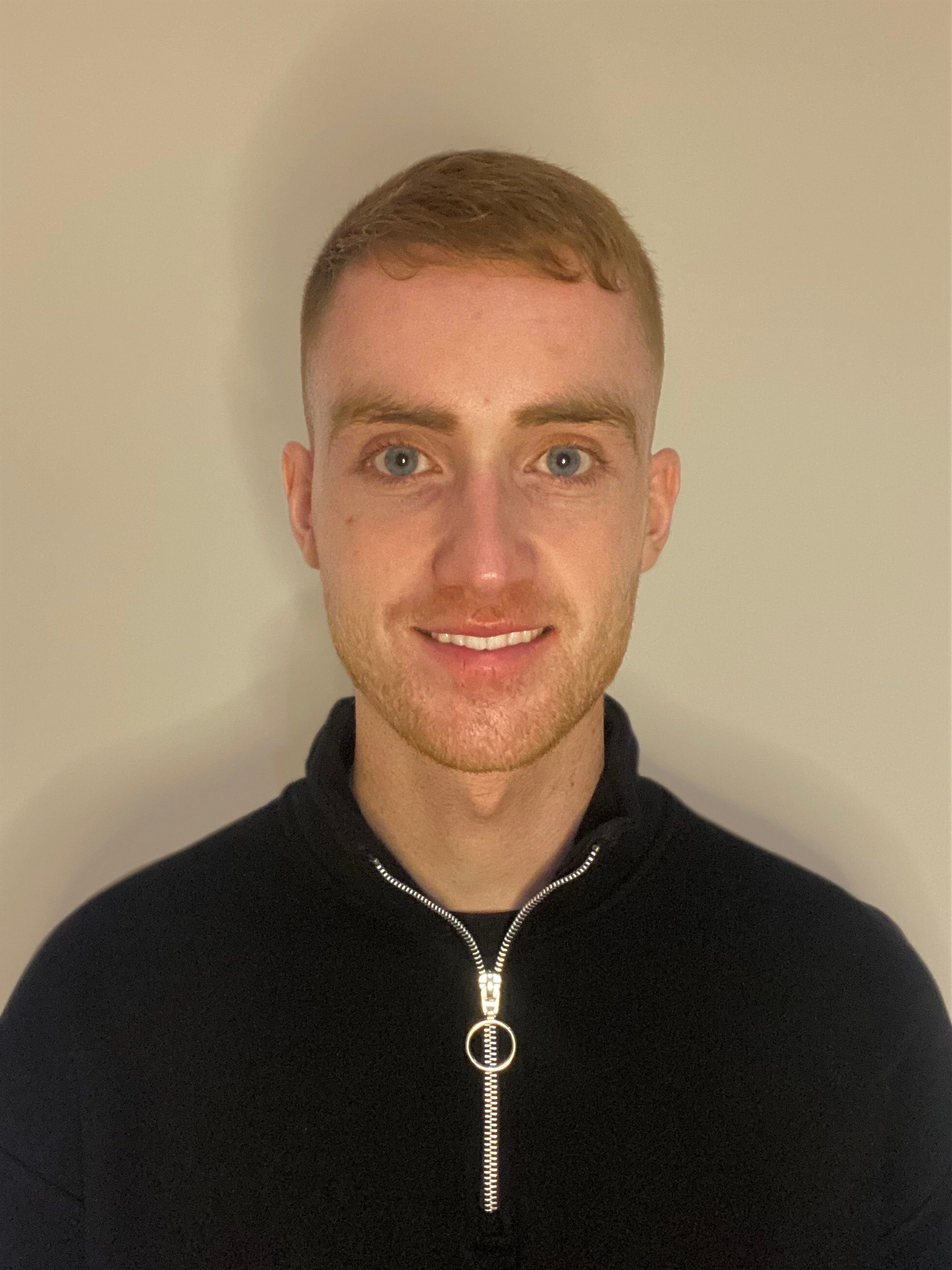
Why Design of Experiments (DOE) is important for biologists
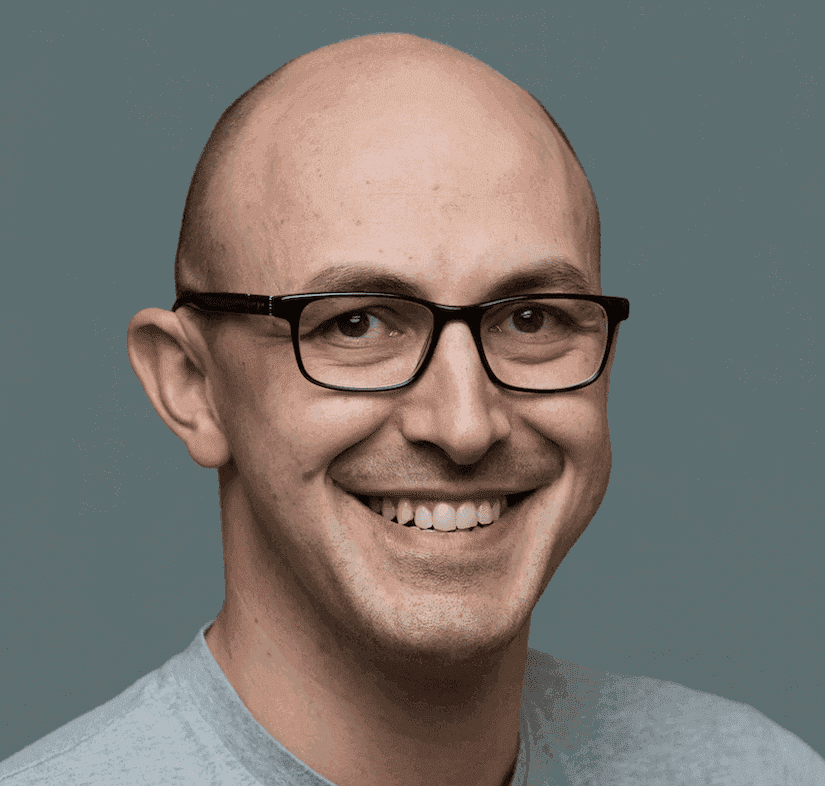
What is Design of Experiments (DOE)?
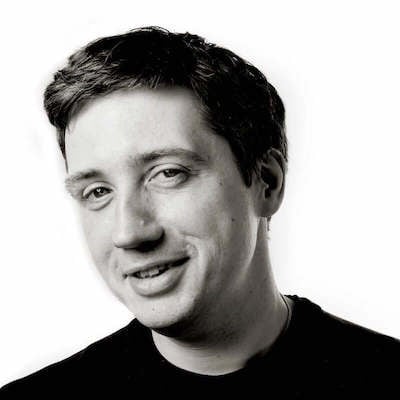