Oxford Biomedica:
83% time savings using Synthace DOE
Oxford Biomedica cut time by 83% across experimental design, planning and execution using Synthace DOE.
Try Synthace DOE Request a demoAt a glance:
- 10-fold increase in vector titre
- 81% reduction in pure error
- 83% time savings
- 32% resource saving
Overview
Oxford Biomedica (OXB) cut time by 83% across experimental design, planning and execution Using Synthace. They've been able to design, plan, and execute a two - iteration DOE optimization and robustness study of their transfection and transduction processes in a dramatically reduced timeframe.
Experiment Profile
- 10-factor simultaneous investigation
- 90-150 experimental runs in a single iteration
- Sophisticated DOE campaign complete in only 2 weeks
Automated DOE
on Synthace
Oxford Biomedica used the Synthace platform to aid planning and execution of a complex multifactorial design space to address questions surrounding yield and robustness of vector particle production.
Given the increase in complexity of experimental design execution afforded by Synthace, Oxford Biomedica wanted to investigate the benefits and value of deviating from a traditional iterative DOE methodology for a quicker optimization strategy.
Manual DOE is impossible
The challenge for Oxford Biomedica was that the process of vector particle production can lead to ineffective as well as active vector particles, and optimal production of active over ineffective vector particles and their ultimate efficacy is largely determined by the respective ratios of the many components contributing to the transfection process. In brief, the process is extraordinarily complex.
This means that a multifactorial design approach is required to address the biological complexity of the system and achieve optimized vector particle production. The company needed an analysis to address the effects of different factors and how they interact. This process is not illuminated by experiments that address one factor at a time, and becomes prohibitively complex to perform using a manual Design of Experiments (DOE) approach if investigating more than five factors.
Results
Oxford Biomedica was able to design, plan, and execute a reduced timeframe two-iteration DOE screening, optimization and robustness study of their transfection and transduction process. The designs in both iterations contained more factors for investigation and more experimental runs than could have been possible if executing manually.
Success metrics:
- A 10-fold increase in vectore titre
- An 81% reduction in pure error
- 83% time savings when compared to their previous experimental process
- 32% resources saved
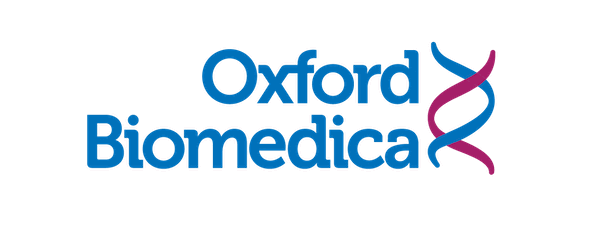
Oxford Biomedica
Oxford Biomedica is the world leader and pioneer in lentiviral vector research, manufacture, and clinical development.
How Oxford Biomedica used Design of Experiments (DOE) to Achieve Improved Titre and Robustness? While also cutting time by 83% across experimental design, planning and execution.